one class SVM は、1 クラスの分類問題に適用するアルゴリズムである。SVM は、一般的に多クラスの分類問題に使われている。例えば、2 クラス分類問題では、片方のクラスの教師ラベルを +1 とし、もう片方のクラスの教師ラベルを -1 として、両者の分離超平面を決定している。そして、未知のサンプルを SVM モデルに代入し、そのサンプルが分離超平面の左側にあれば +1、そうでなければ -1 と予測できる。
多クラス分類に適用する SVM に対して、one class SVM は、すべてのサンプルの教師ラベルを +1 とし、原点の教師ラベルを -1 として、分離超平面を決定している。このように決定された分離超平面を利用することで、未知のサンプルが分離超平面の原点側にあるか、それとも反対側になるのかを見て、外れ値かどうかを判定している。
scikit-learn で one class SVM モデルを構築するときは、OneClassSVM
関数を利用する。
import numpy as np
import matplotlib.pyplot as plt
import matplotlib.font_manager
from sklearn import svm
xx, yy = np.meshgrid(np.linspace(-5, 5, 500), np.linspace(-5, 5, 500))
# train data
X = 0.3 * np.random.randn(100, 2)
X_train = np.r_[X + 2, X - 2]
# test data (normal)
X = 0.3 * np.random.randn(20, 2)
X_test = np.r_[X + 2, X - 2]
# test data (abnormal)
X_outliers = np.random.uniform(low=-4, high=4, size=(20, 2))
# fit the model
clf = svm.OneClassSVM(nu=0.2, kernel='rbf', gamma=0.1)
clf.fit(X_train)
y_pred_train = clf.predict(X_train)
y_pred_test = clf.predict(X_test)
y_pred_outliers = clf.predict(X_outliers)
# plot the line, the points, and the nearest vectors to the plane
Z = clf.decision_function(np.c_[xx.ravel(), yy.ravel()])
Z = Z.reshape(xx.shape)
plt.figure(figsize=(4, 4), dpi=150)
plt.title('Outlier detection')
plt.contourf(xx, yy, Z, levels=np.linspace(Z.min(), 0, 7), cmap=plt.cm.PuBu)
a = plt.contour(xx, yy, Z, levels=[0], linewidths=2, colors='darkred')
plt.contourf(xx, yy, Z, levels=[0, Z.max()], colors='palevioletred')
s = 40
b1 = plt.scatter(X_train[:, 0], X_train[:, 1], c='white', s=s, edgecolors='k')
b2 = plt.scatter(X_test[:, 0], X_test[:, 1], c='blueviolet', s=s, edgecolors='k')
c = plt.scatter(X_outliers[:, 0], X_outliers[:, 1], c='gold', s=s, edgecolors='k')
plt.axis('tight')
plt.xlim((-5, 5))
plt.ylim((-5, 5))
plt.legend([a.collections[0], b1, b2, c],
['learned frontier', 'training data', 'test data (normal)', 'test data (abnormal)'],
loc="upper left",
prop=matplotlib.font_manager.FontProperties(size=11))
学習データで決定された超平面は、次の図の赤色の曲線(輪)で囲まれている部分になる。
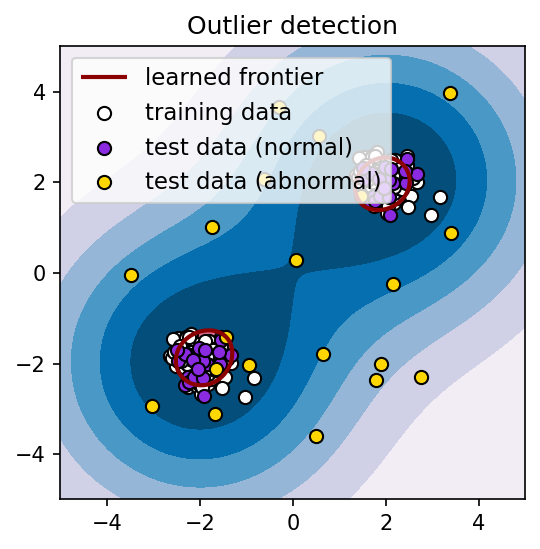
ハイパーパラメーターである nu
の値を変更することにより、外れ値の判定を強めたり、弱めたりすることができる。例えば、上記のコード中において、nu = 0.002
と値を小さくすると、分離超平面は次のようになる。
clf = svm.OneClassSVM(nu=0.2, kernel='rbf', gamma=0.1)
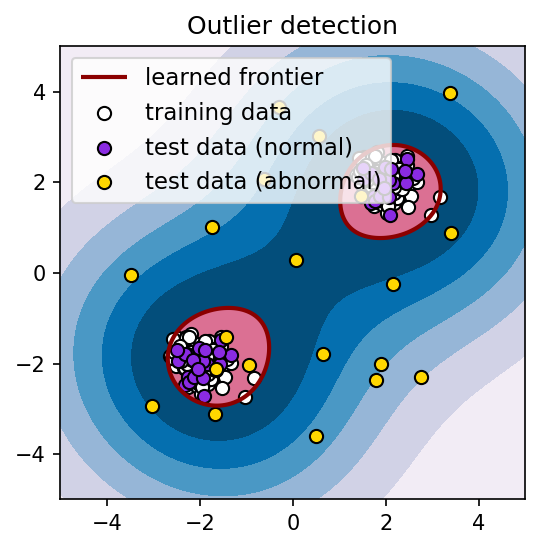
References
- One-class SVM with non-linear kernel (RBF). HTML