ROC 曲線は、モデルの性能評価に使われている。ROC 曲線は、予測結果から計算される false positive rate を横軸に、true positive rate を縦軸に点をプロットし、それを線でつないだグラフである。点の数が多くなると、線が滑らかな曲線のように見えるので、曲線と呼ばれている。
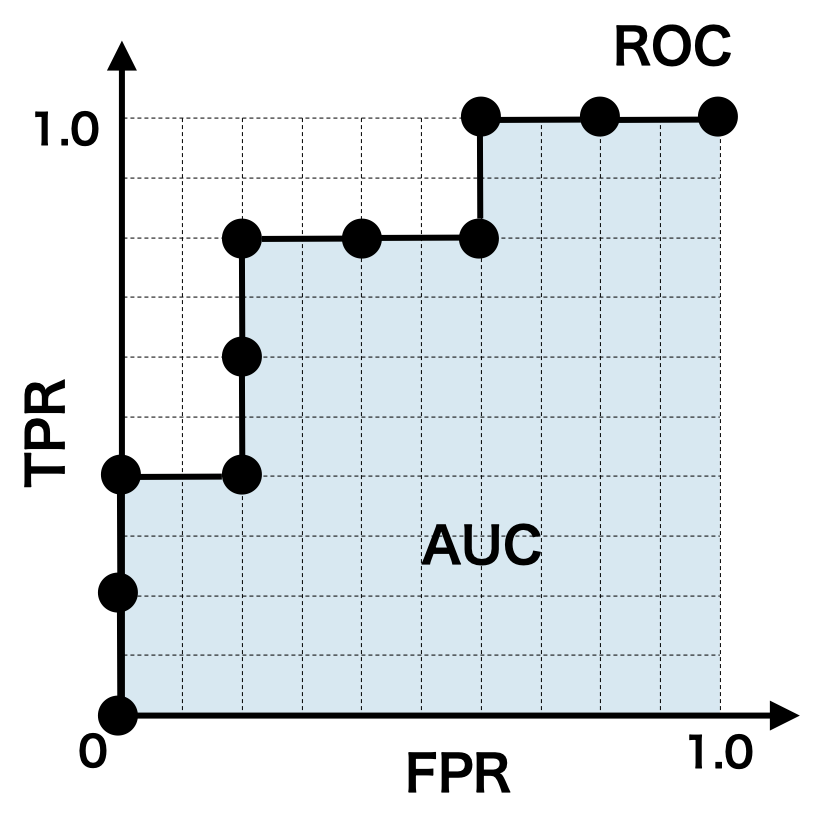
予測モデルが出力するスコアに基づいて、スコアの高い順にデータを並べ替える。次に、スコアに対して閾値を設けて、閾値を超えた場合に positive、閾値以下の場合に negative と判定する。判定結果と教師ラベルを比較し TPR および FPR を計算する。以下に ROC 曲線を描く例を示す。
まず、予測結果をスコア順に並べて、最も高いスコアの上に閾値を置く。閾値以下はすべて negative であるので、この場合、予測結果は TN または FN しかない。これらの値を元に TPR および PFR を計算し、それを座標上にプロットする。
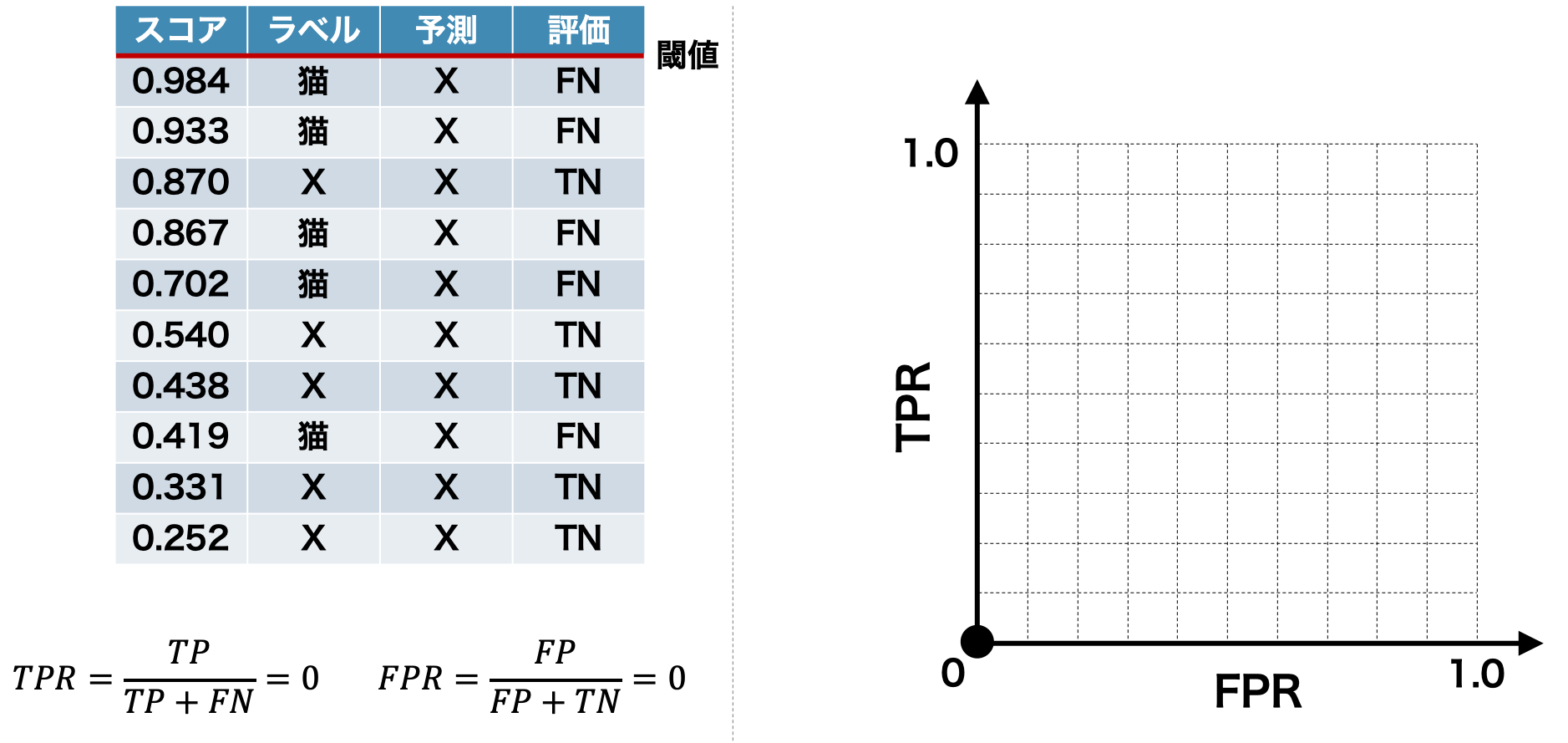
次に、閾値を最高スコアと 2 番目に大きいスコアの間に移動する。そして、同様にして TPR および FPR を計算して、座標上にプロットする。
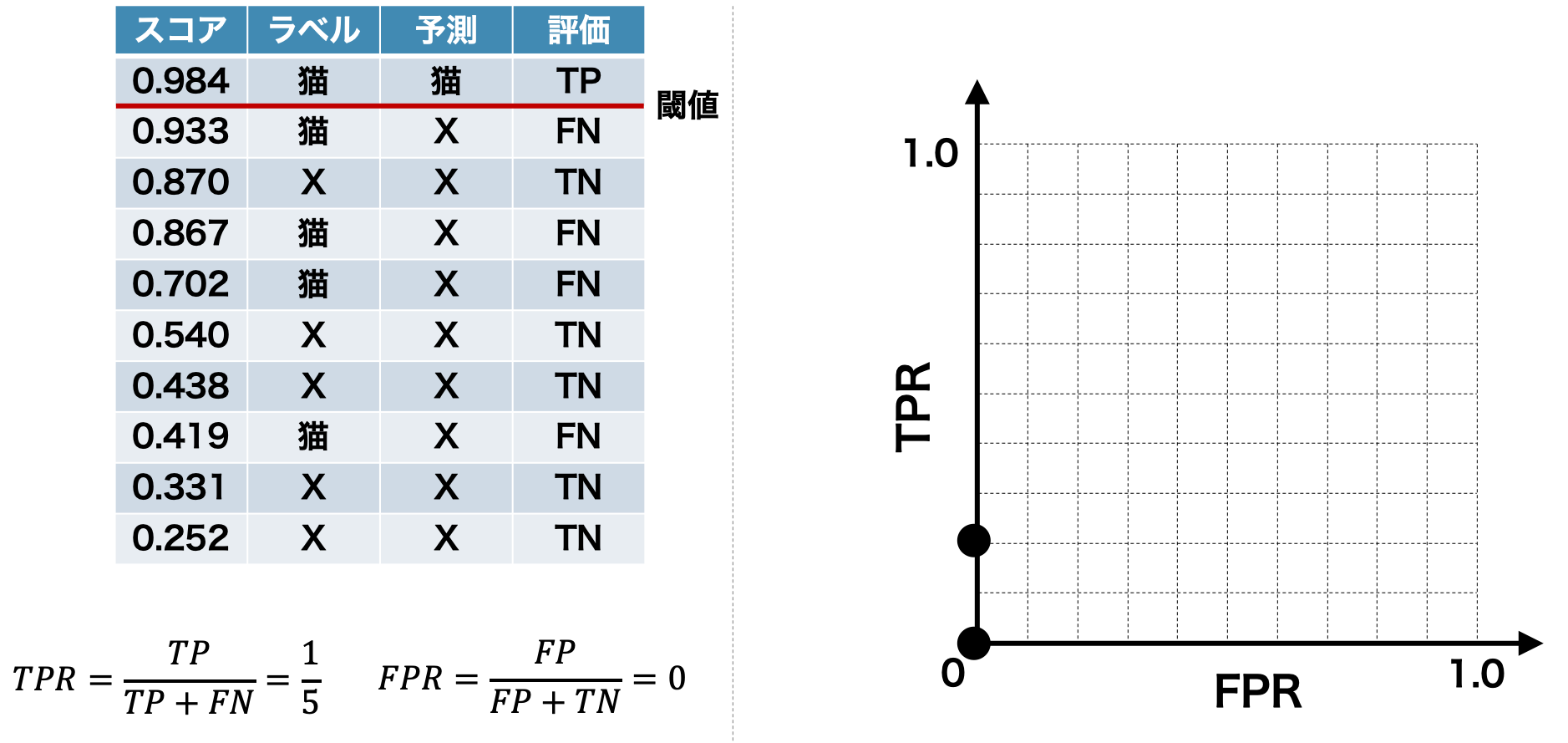
このように閾値を少しずつずらしながら、点をプロットする。
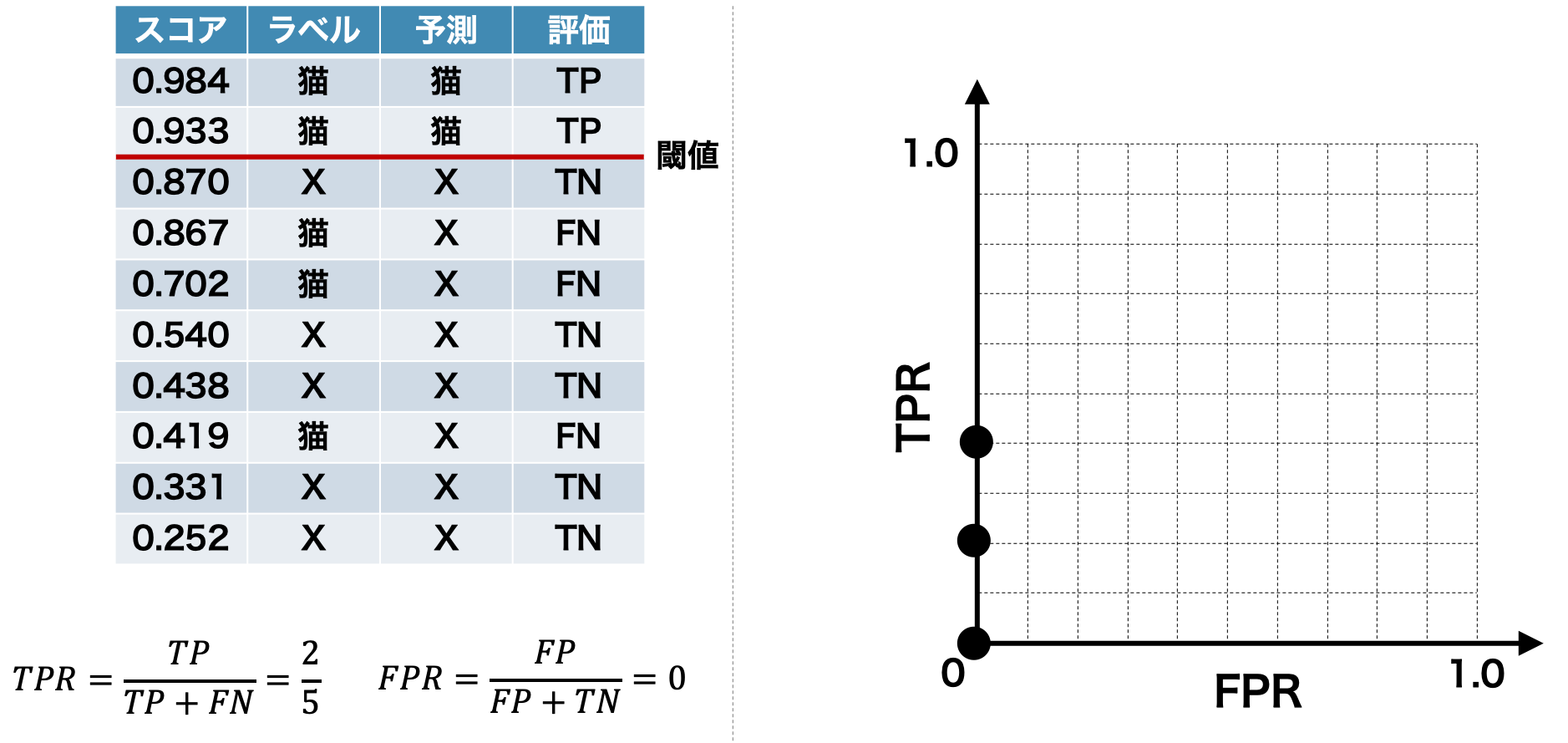
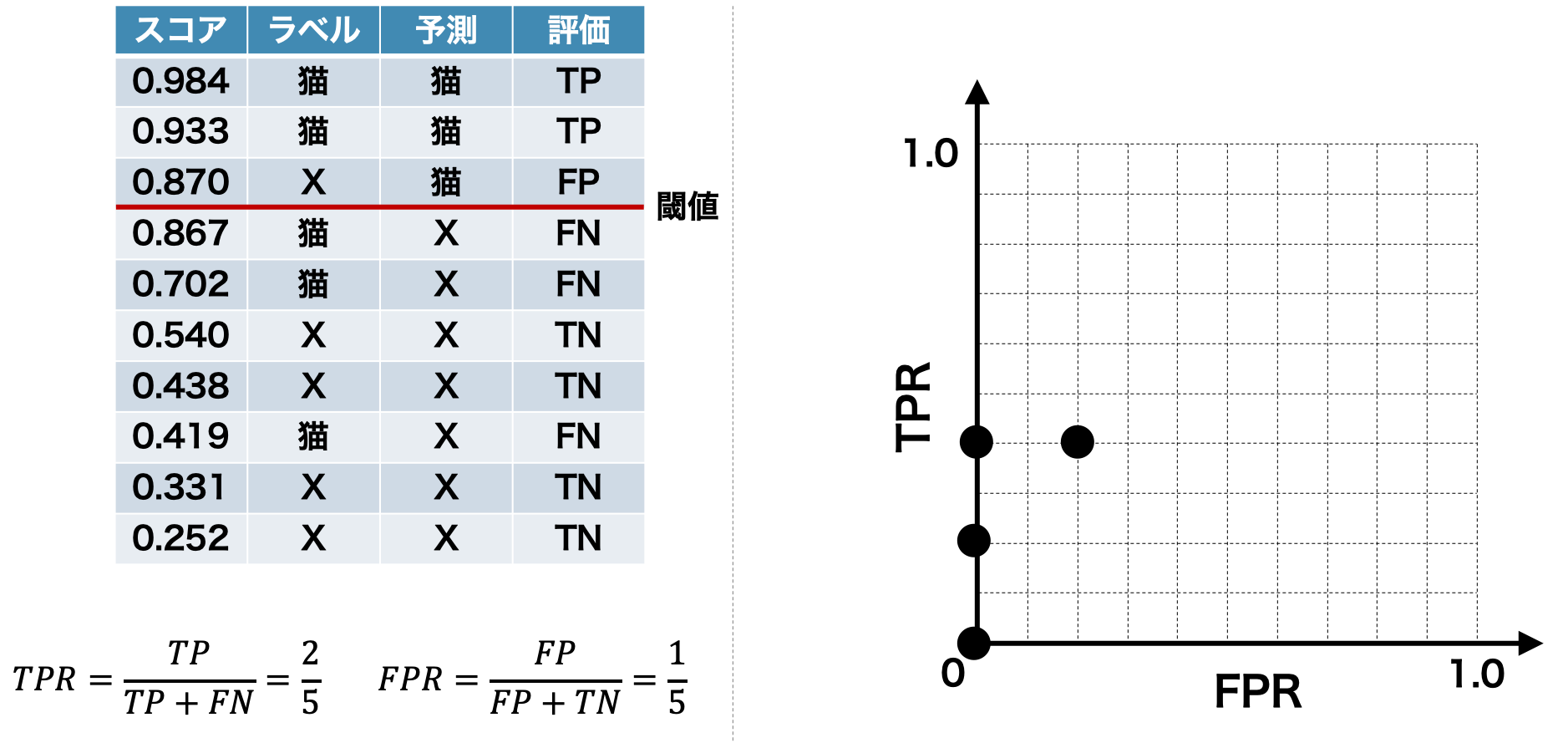
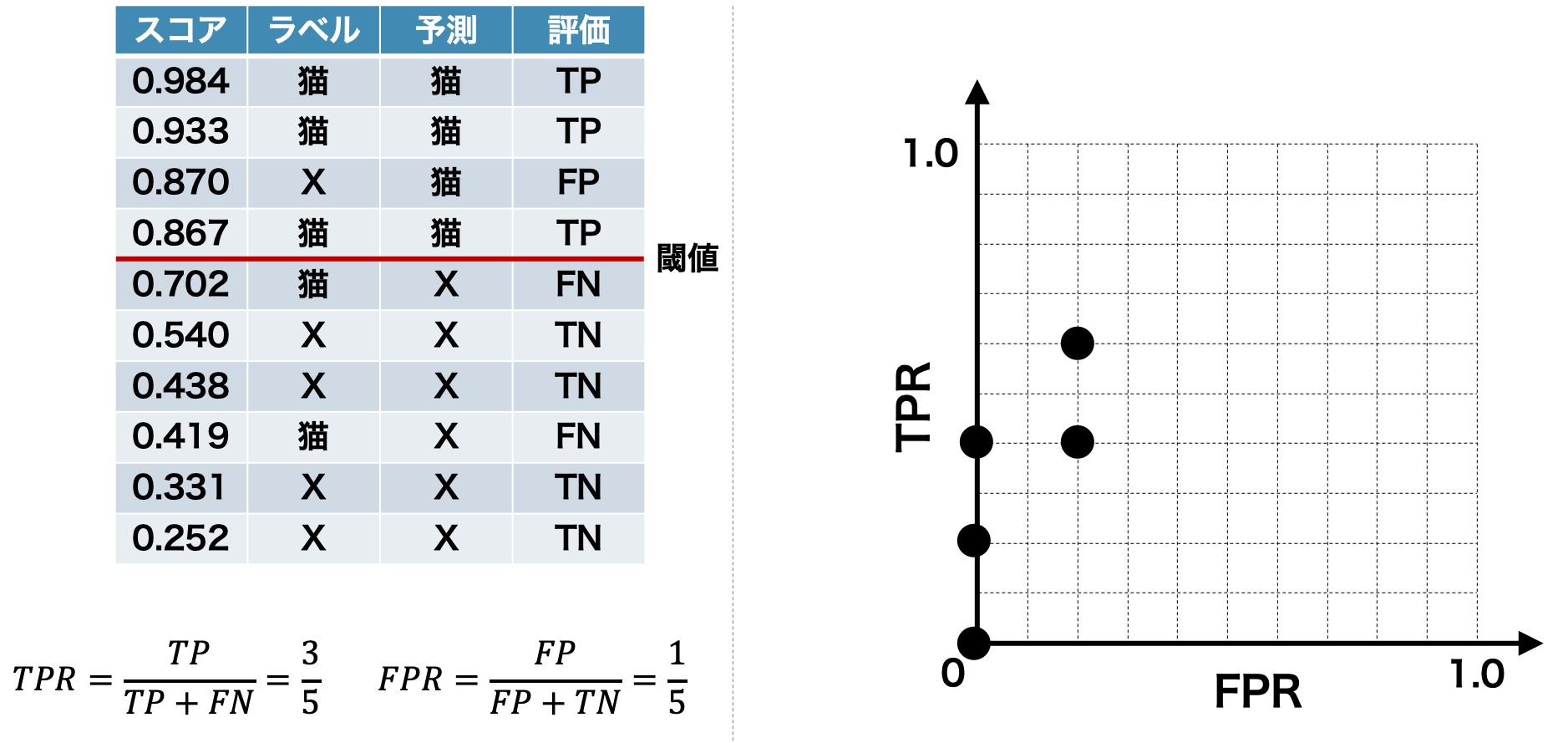
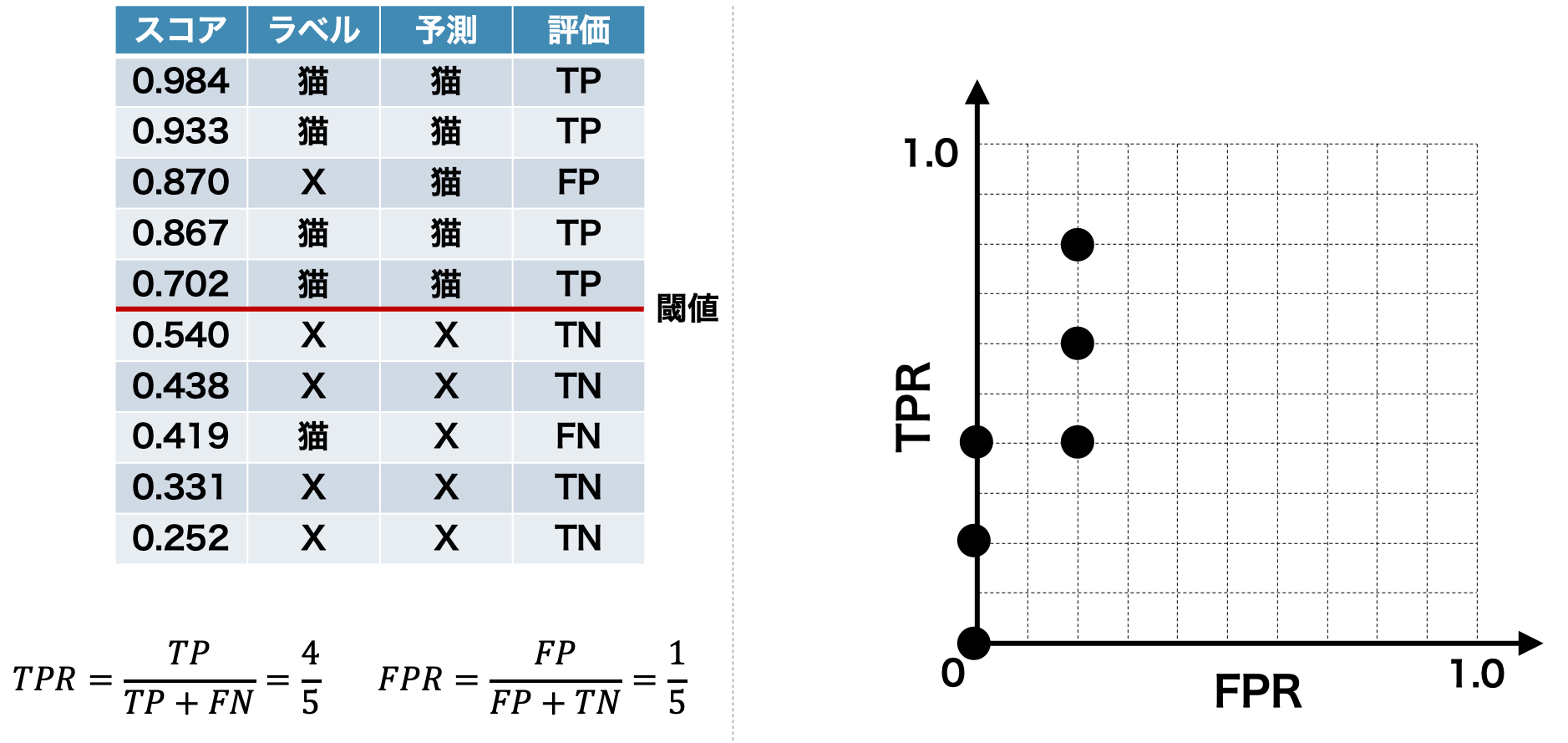
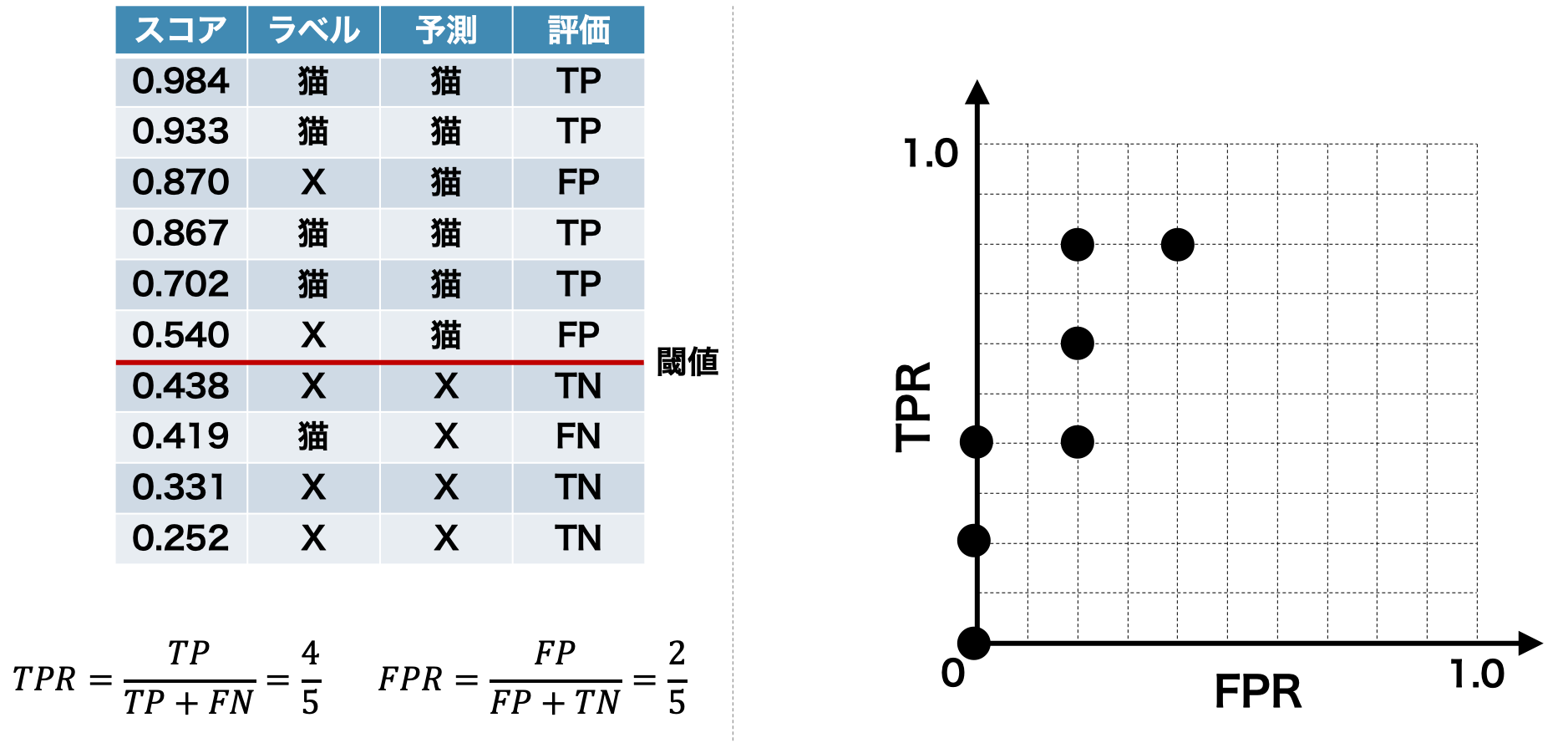
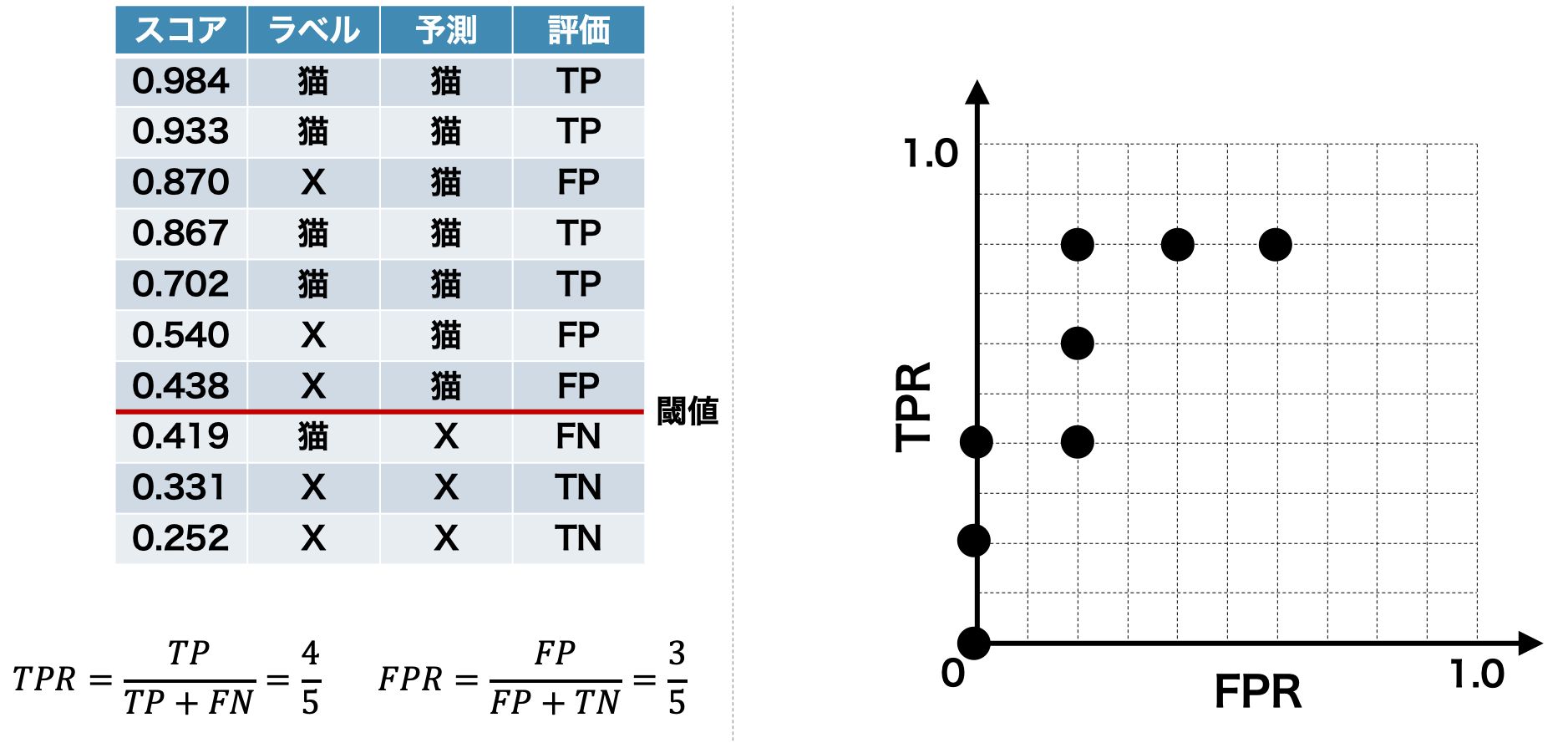
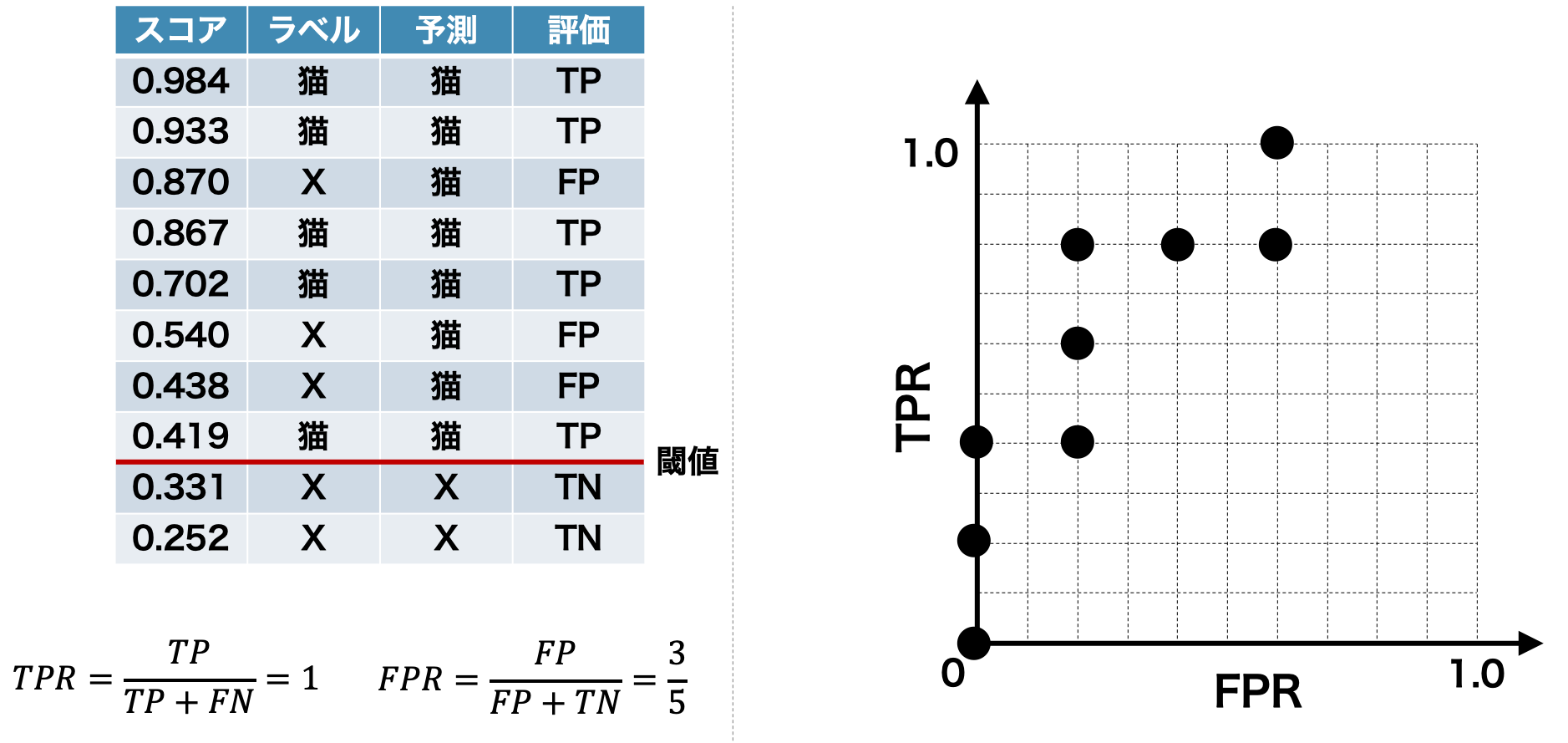
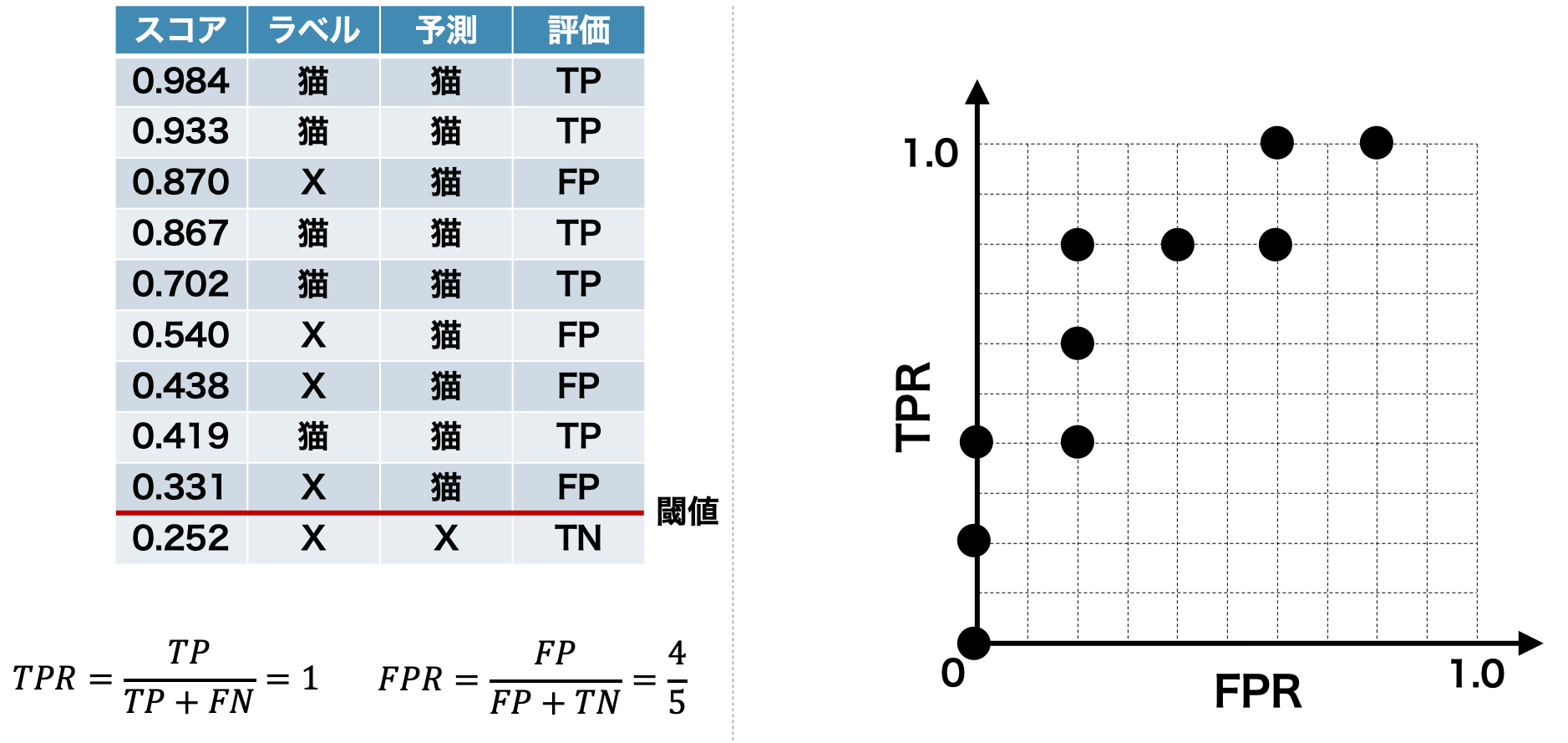
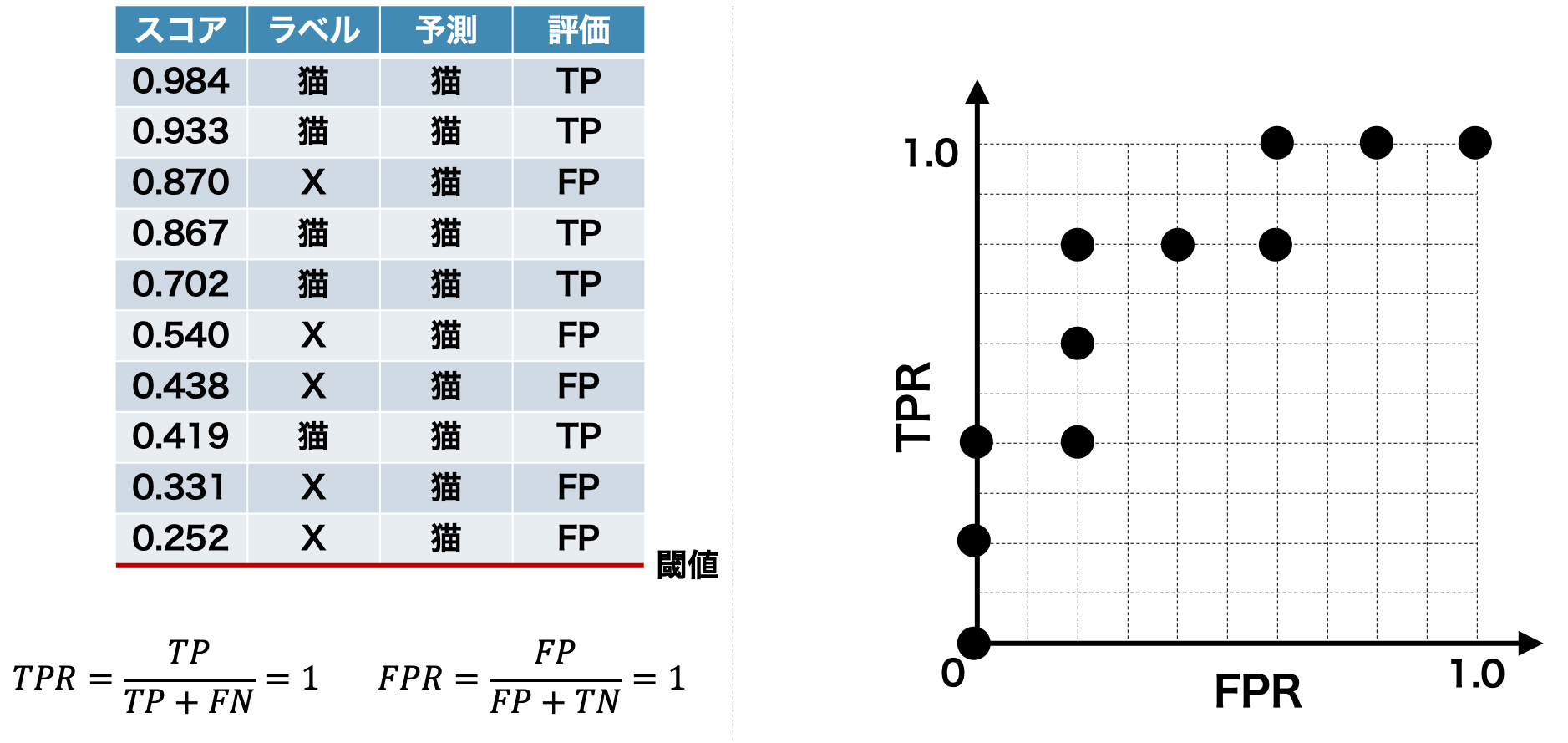
最後にすべての点を線で結ぶことで、ROC 曲線が描かれる。ROC 曲線の下の部分の面積を AUC とよび、AUC が 1 に近づくほどモデルの性能が良いとされる。
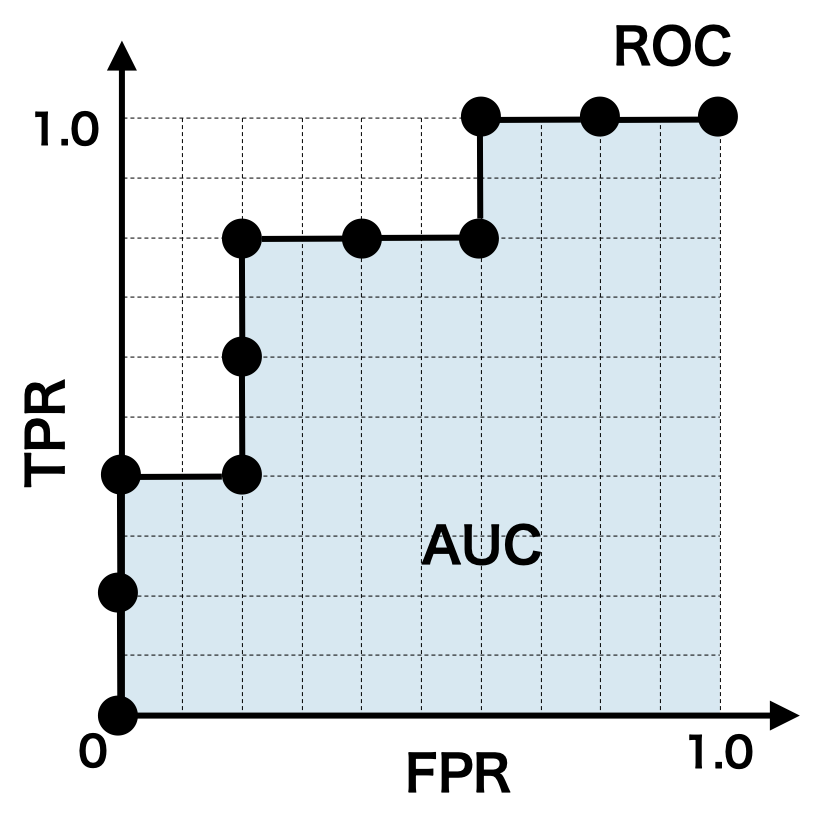
scikit-learn で ROC 曲線を描く方法
次のコードは、乳がんデータの疾患に対する予測モデルを SVM とロジスティック回帰の 2 つの方法で作成し、2 つのモデルの ROC 曲線を描く例である。ROC 曲線の座標を計算するためのスコアが必要なため、SVM モデルを作成するときに、probability=True
を指定する必要がある。
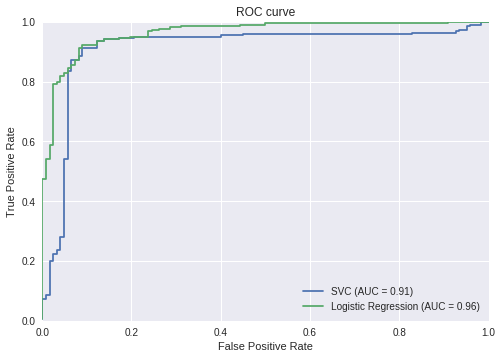